Srivastava Group – Research
Research focus
Our research group seeks to apply novel Deep Learning and Machine Learning based algorithms on seismic signals to improve Earthquake Early Warning System (EEWs) and Seismic Signal Analysis. Additionally, by implementing distinct Artificial Intelligence-driven models, supported by the statistical analysis based on classical models we are also trying to better understand the pattern associated with Seismic stress release. Earthquakes are inevitable and considered extremely difficult to predict. Analyzing and interpreting the ever-increasing abundant seismological dataset is the most time-consuming and is a herculean task. The implementation of Artificial Intelligence powered by Deep Learning algorithms for earthquake signal analysis has the potential to out-perform classical approaches such as STA/LTA.
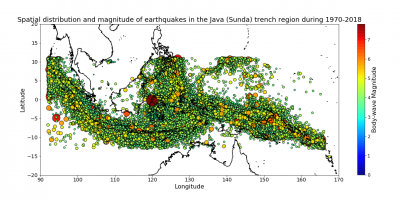
Seismic Event Detection and Seismic phase picking are the two most crucial tasks for seismologists. Especially in the case of low signal-to-noise ratio, small magnitude seismic events are extremely hard to be detected by classical methods and researchers try to identify such microearthquakes manually. We are working on a simple yet powerful Deep Learning architecture based on an encoder-decoder algorithm comprised of 1-Dimensional Convolutional Layers with skip connections and Self- & Multi-Attention mechanism. This architecture is currently being applied and tested on various seismic datasets from different regions to check its performance. Owing to its higher sensitivity, this model is expected to perform better than the classical approach.
Furthermore, we are attempting to improve the existing EEWs models by reducing the length of data used as input as well as the needed number of stations. Various combinations of Convolutional Neural Networks and Bi- & Uni-directional Long-Short Term Memory and Gated Recurrent Unit are being tried and tested to obtain the best performing model with optimal hyperparameters. This study is likely to help seismologists in issuing quicker warnings, thus providing increased time window for evacuation to the local authorities.