Bertschinger Group – Research
Information theory of volatility dynamics
The statistical description and modeling of volatility plays a prominent role in econometrics, risk management and finance. Stochastic volatility models describe volatility as a hidden random process that in turn effects the size of observed market returns. Using information theory we have studied the following question: How much information about volatility can be obtained from observed prices alone?
Using tools from information theroy we could prove that for a wide class of stochastic volatility models information about volatility is inherently limited for fundamental theoretic reasons. Thus, reliable estimates of volatility require either high-frequency price data or access to option prices.
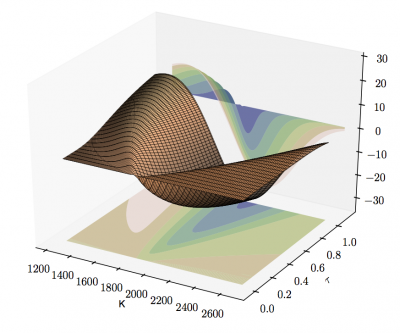
Statistical analysis of econophysics models
Standard econometric models of volatility, such as GARCH or stochastic volatility models, provide a purely phenemonelogical description of market dynamics. In contrast, agent-based models from econophysics assume that markets consist of a large number of possibly hetereogeneous traders. In this view, observed price dynamics then emerge from collective actions of many traders, individually following mechanistic rules capturing basic psychological processes such as herding behavior. Thus, highlighting potential mechanisms that could drive the dynamics of volatility.
Up to now, such models are mostly compared on qualitative grounds, i.e. in terms of their ability to replicate global statistical properties -- stylized facts -- of asset prices. Here, utilizing modern tools from machine learning we fit several models and provide rigorous quantitative comparisons with standard econometric models.
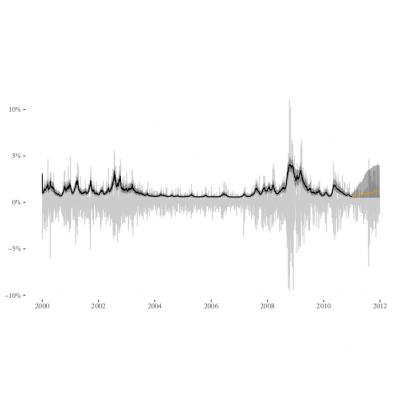
Gaussian processes for portfolio optimization
Different asset classes, e.g. stocks of companies from several sectors, react differently, yet not independently, to general market downturns. Commonly, such correlations between stocks are described by factor models. Here, it is assumed that just a few risk factors effect all stocks, albeit each to a different degree. By using Gaussian processes, i.e. modern tools from machine learning, we could extend these models to express non-linear dependencies between stock returns. This not only leads to much better statistical models for stock correlations, but in turn, allows to build investment portfolios achieving impressive returns.
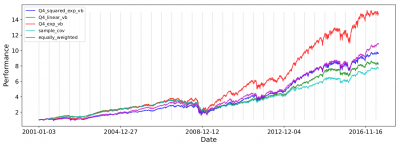
Network contagion in financial markets
The ever increasing complexity of financial interconnections poses new threats to financial stability. Especially the latest financial crisis has revealed the potential of cascading failures that could lead to the break-down of the global financial system. In stylized models of financial markets, we investigate several contagion mechanisms, e.g. direct spreading via counter parties defaulting or indirect spreading via fire sales of commonly held financial assets, that have been proposed in the literature. Combining computer simulations with theoretical analysis we investigate the cost and effectiveness of different bail-out strategies.
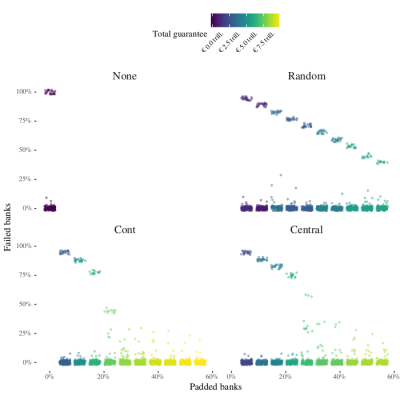
Publications
Publication list at Google Scholar